GOTO, D., SCHUTGENS, N.A.J., OIKAWA, E., TAKEMURA, T., NAKAJIMA, T.
CGER Reports
CGER’s Supercomputer Monograph Report Vol. 23
Summary
Aerosols have a great impact on not only air pollution on a local scale but also climate change on a global scale. They can modulate shortwave and longwave radiative fluxes in the atmosphere; thus they also affect the retrieving results of other species such as CO2 from satellites. To properly estimate the distributions of aerosols, an improvement of aerosol transport models is required. We have developed a global aerosol transport model, which was originally provided by Takemura et al. (2000), named a Spectral Radiation-Transport Model for Aerosol Species (SPRINTARS). The aerosol module has been coupled with the Model for Interdisciplinary Research on Climate (MIROC by Watanabe et al., 2010) and recently with the Non-hydrostatic Icosahedral Atmospheric Model (NICAM by Tomita and Satoh, 2004). The module has been compared with various observations including in-situ measurements, remote sensing through satellites, skyradiometer, and lidar, and other aerosol transport models through various model comparisons such as the AeroCom international project. Such comparisons help us to continuously improve the model. Under the NIES-SX supercomputer project, we have been comparing and improving the SPRINTARS for 10 years. Our major research papers are described in Chapters 1 and 2 (Goto et al., 2011; Oikawa et al., 2013).
Chapter 1 provides an improvement of sulfur chemistry in the SPRINTARS module of MIROC by comparisons with other global models focusing on the relationship between sulfate burden and direct aerosol radiative forcing (DARF) due to sulfate (Figure 1), as well as on the sulfur budget. Chapter 2 provides a new validation method of global aerosol transport models using Cloud-Aerosol Lidar with Orthogonal Polarization (CALIOP) on space-borne Cloud-Aerosol Lidar and Infrared Pathfinder Satellite Observation (CALIPSO), which focuses on the vertical distribution of aerosols under clear-sky/cloudy-sky conditions and its impacts on aerosol radiative forcing (Figure 2). In addition, we have developed a data assimilation method for aerosols to provide more accurate results of aerosol distributions using satellite-based observations (Schutgens et al., 2010a).
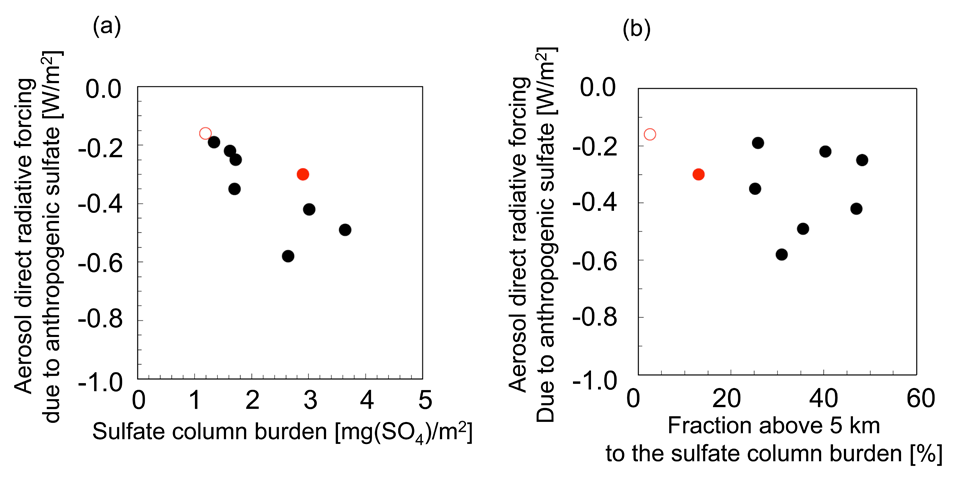
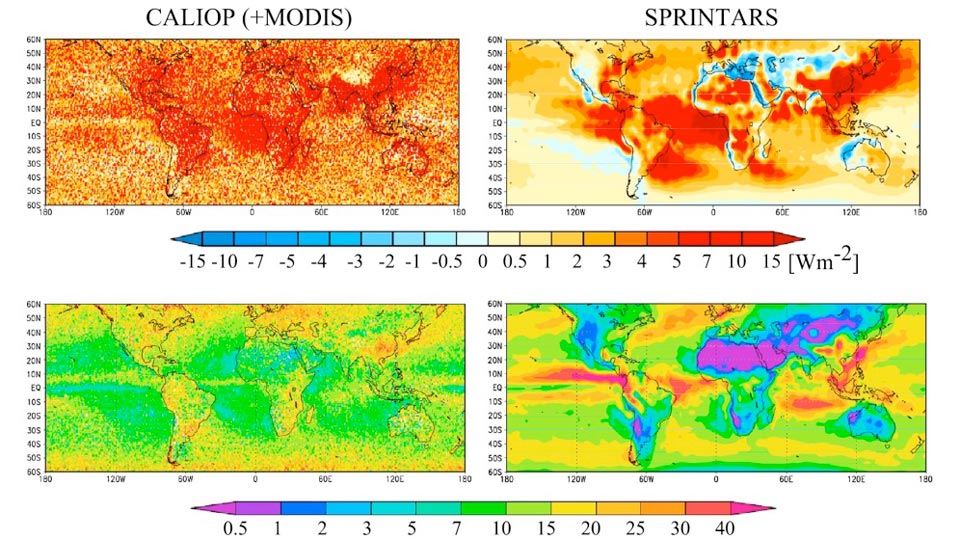
In Chapter 3, an aerosol assimilation system using an ensemble Kalman Filter method is introduced. Unlike other assimilation schemes, the ensemble allows realistic, spatially and temporally variable model covariances. As the analyzed variables are mixing ratios (prognostic variables of the aerosol transport model), there is no need for the extra assumptions required by previous assimilation schemes analyzing aerosol optical thickness (AOT). The assimilation system was validated through various sensitivity experiments (Figure 3 and Schutgens et al., 2010a, 2010b) and was extended to a Kalman smoother method to estimate emission inventories (Schutgens et al., 2012). A successful assimilation system can be applied for supporting retrievals from satellites such as the Greenhouse gases Observing SATellite (GOSAT). Originally, the MIROC-SPRINTARS was used as reference data for processing level 2 datasets of shortwave Infrared (SWIR) by the Fourier Transform Spectrometer (FTS). Very recently, the processing system has been tested by a new assimilation system coupled with MIROC-SPRINTARS to reprocess higher-level products from GOSAT.
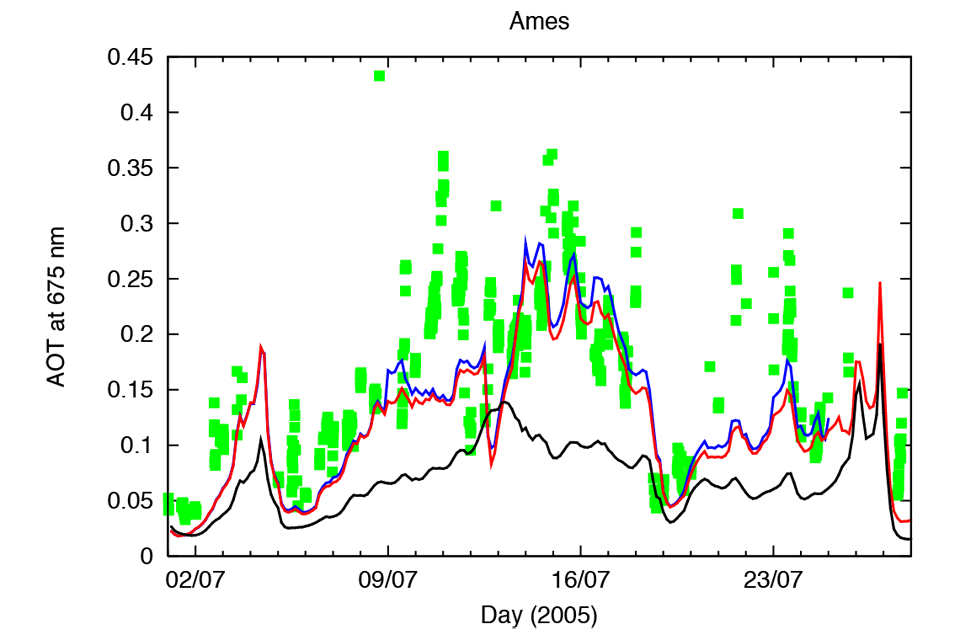
References
The present report is based on the following papers:
- Goto D., Nakajima T., Takemura T., Sudo K. (2011) A study of uncertainties in the sulfate distribution and its radiative forcing associated with sulfur chemistry in a global aerosol model. Atmospheric Chemistry and Physics, 11, 10889-10910.
- Oikawa E., Nakajima T., Inoue T., Winker D. (2013) A study of the shortwave direct aerosol forcing using ESSP/CALIPSO observations and GCM simulation. Journal of Geophysical Research, 118, 3687-3708, doi:10.1002/jgrd.50277.
- Schutgens N.A.J., Miyoshi T., Takemura T., Nakajima T. (2010a) Applying an ensemble Kalman filter to the assimilation of AERONET observations in a global aerosol transport model. Atmospheric Chemistry and Physics, 10, 2561-2576.
Other references are as follows:
- Schulz M., Textor C., Kinne S., Balkanski Y., Bauer S., Bertsen T., Berglen T., Boucher O., Dentener F., Guibert S., Isaksen I.S.A., Iversen T., Koch D., Kirkevag A., Liu X., Montanaro V., Myhre G., Penner J. E., Pitari G., Reddy S., Seland O., Stier P., Takemura T. (2006) Radiative forcing by aerosols as derived from the AeroCom present-day and pre-industrial simulations, Atmospheric Chemistry and Physics, 6, 5225-5246.
- Schutgens N.A.J., Miyoshi T., Takemura T., Nakajima T. (2010b) Sensitivity tests for an ensemble Kalman filter for aerosol assimilation, Atmospheric Chemistry and Physics, 10, 6583-6600.
- Schutgens N.A.J., Nakata M, Nakajima T. (2012) Estimating Aerosol Emissions by Assimilating Remote Sensing Observations into a Global Transport Model. Remote Sensing, 4(11), 3528-3543, doi:10.3390/rs4113528.
- Takemura T., Okamoto H., Maruyama Y., Numaguti A., Higurashi A., Nakajima T. (2000) Global three-dimensional simulation of aerosol optical thickness distribution of various origins, Journal of Geophysical Research, 105, 17853–17873.
- Textor C., Schulz M., Guibert S., Kinne S., Balkanski Y., Bauer S., Berntsen T., Berglen T., Boucher O., Chin M., Dentener F., Diehl T., Easter R., Feichter H., Fillmore D., Ghan S., Ginoux P., Gong S., Grini A., Hendricks J., Horowitz L., Huang P., Isaksen I., Iversen I., Kloster S., Koch D., Kirkevåg A., Kristjansson J. E., Krol M., Lauer A., Lamarque J. F., Liu X., Montanaro V., Myhre G., Penner J., Pitari G., Reddy S., Seland Ø., Stier P., Takemura T., Tie X. (2006) Analysis and quantification of the diversities of aerosol life cycles within AeroCom, Atmospheric Chemistry and Physics, 6, 1777–1813.
- Tomita H., Satoh M. (2004) A new dynamical framework of non-hydrostatic global model using the icosahedral grid, Fluid Dynamics Research, 34, 357–400, doi: 10.1016/j.fluiddyn.2004.03.003.
- Watanabe M., Suzuki T, O’ishi R, Komuro Y, Watanabe S, Emori S, Takemura T, Chikira M, Ogura T, Sekiguchi M, Takata K, Yamazaki D, Yokohata T, Nozawa T, Hasumi H, Tatebe H, Kimoto M (2010) Improved climate simulation by MIROC 5: mean states, variability, and climate sensitivity, Journal of Climate, 23, 6312-6335, doi: 10.1175/2010JCLI3679.1.